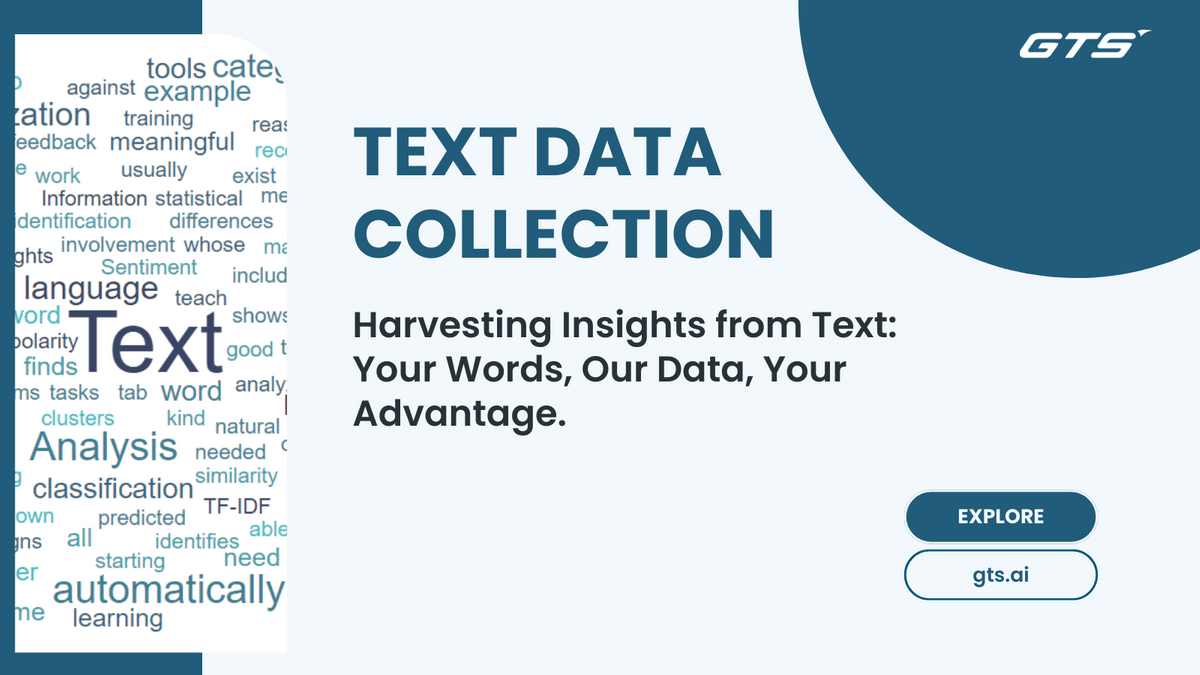
Introduction
In an age where data reigns supreme, and artificial intelligence (AI) drives innovation across industries, the role of Text Data Collection in the world of machine learning cannot be overstated. From virtual assistants and recommendation systems to sentiment analysis and language translation, text data plays a pivotal role in shaping the capabilities and possibilities of AI.
This blog post will take you on a journey deep into the heart of AI-enhanced machine learning, exploring the crucial role of text data collection and its profound impact on the advancement of AI applications.
The Text Data Revolution
Our journey begins by acknowledging the transformative force of AI in our lives. AI has revolutionized how we interact with technology, from voice-controlled assistants like Siri and Alexa to intelligent recommendation systems on our favorite streaming platforms. Behind these innovations is a wealth of text data, fueling AI's ability to understand and respond to human language.
Text data forms the backbone of many AI applications, enabling machines to comprehend, analyze, and generate human-like text. From social media posts to news articles and customer reviews, this data source offers a rich tapestry of human expression that machines can learn from and respond to.
To truly appreciate the significance of text data in AI, let's delve deeper into the nature of this valuable resource.
The Building Blocks: What Is Text Data?
Defining Text Data
Text data, as the name suggests, is simply data represented in textual form. It encompasses written words and characters, often found in documents, emails, books, social media posts, and more. Text data can be classified into two main categories: structured and unstructured.
Structured text data refers to information that is organized in a specific format, making it relatively easy to analyze. Think of a database containing customer names and their corresponding email addresses. This is structured text data.
Unstructured text data, on the other hand, is far more complex. It lacks a predefined structure, and its content can vary greatly. Examples of unstructured text data include social media posts, customer reviews, and articles. Unstructured text data poses unique challenges and opportunities for AI and machine learning.
The Challenges and Opportunities Presented by Text Data

Unstructured text data, with its rich diversity and variability, poses several challenges for AI and machine learning. Unlike structured data, where the format is consistent and the relationships are clearly defined, unstructured text data requires the ability to interpret context, sentiment, and nuance.
Despite these challenges, the opportunities presented by text data are immense. Unstructured text data holds a wealth of information, insights, and hidden patterns waiting to be uncovered. This text data is the key to understanding the ever-evolving language and thought of human beings.
To harness the power of text data for AI-enhanced machine learning, we must embark on a journey that begins with data collection.
Text Data Collection: The Heart of AI
The saying "garbage in, garbage out" is especially true when it comes to AI and machine learning. To create robust and accurate AI models, the data we feed them must be of high quality. This is where text data collection comes into play.
The Importance of High-Quality Data
High-quality text data is the cornerstone of any successful AI application. It's the difference between a chatbot that understands and responds to user queries effectively and one that constantly misunderstands and frustrates users. To achieve high quality, data must be accurate, relevant, and up-to-date.
Strategies for Text Data Collection
1. Web Scraping
Web scraping is a technique used to extract data from websites. It allows organizations to gather large volumes of text data from the internet, which can be invaluable for training machine learning models. Whether you're interested in monitoring news articles, social media posts, or online reviews, web scraping can provide the data you need.
2. Data Labeling and Annotation
Text data often needs to be labeled or annotated to make it useful for machine learning. For example, if you're training a sentiment analysis model, you'll need text data labeled as positive, negative, or neutral. Human annotators are often employed to perform this task, ensuring that the data is correctly labeled for training purposes.
3. User-Generated Content
User-generated content, such as customer reviews, forum discussions, and social media posts, offers a wealth of text data. This data is invaluable for training AI models in tasks like sentiment analysis, content recommendation, and social listening.
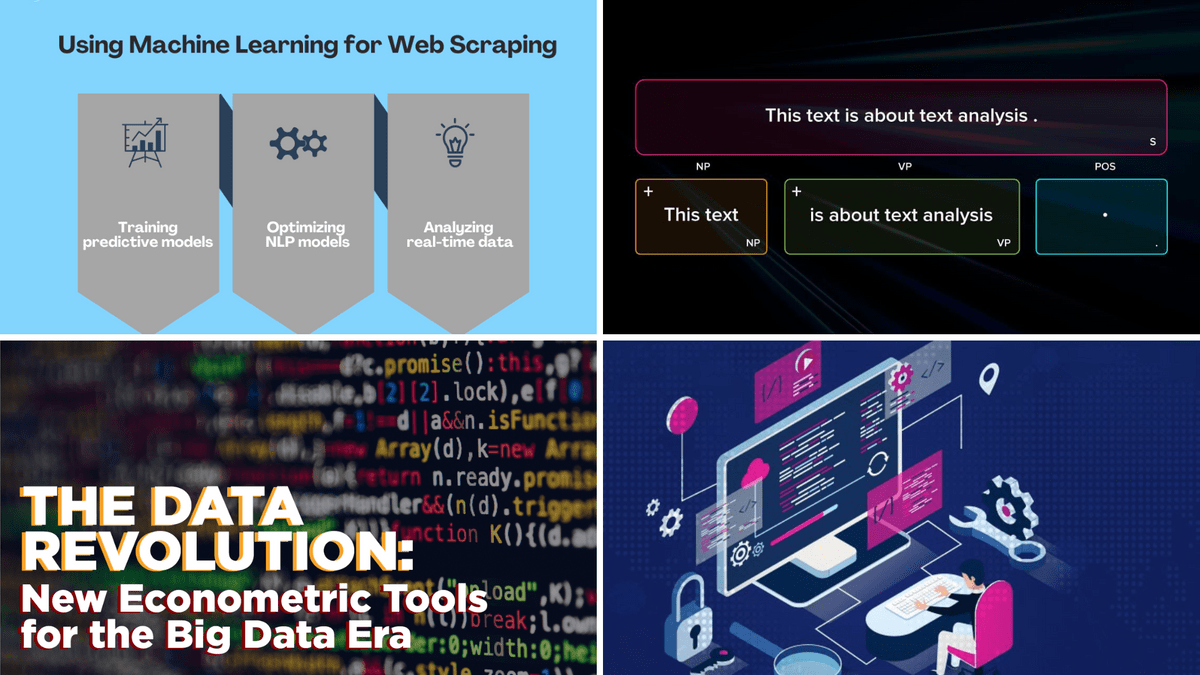
Text Data in Action: Applications of AI-Enhanced Machine Learning
Text data is a versatile resource that underpins a wide array of AI applications. Let's take a closer look at some of these applications.
Natural Language Processing (NLP)
1. Sentiment Analysis: Sentiment analysis, also known as opinion mining, is a popular NLP application. It involves determining the sentiment expressed in a piece of text, whether it's positive, negative, or neutral. Businesses use sentiment analysis to gauge customer feedback and sentiment on social media, helping them make informed decisions.
2. Language Translation: Machine translation has come a long way, thanks to NLP. Services like Google Translate use AI to translate text from one language to another, making cross-lingual communication more accessible.
3. Chatbots and Virtual Assistants: Chatbots and virtual assistants are becoming increasingly sophisticated in their ability to understand and respond to natural language. They rely on NLP to provide users with accurate and helpful information.
Text Data's Impact on Machine Learning Models
Now that we've seen the applications of text data, let's explore how it impacts machine learning models.
How Text Data Enhances Model Training
Text data augments the training of machine learning models in various ways. By exposing models to vast amounts of text data, they learn the intricacies of language, including context, semantics, and grammar. This knowledge is invaluable when models need to understand and generate text, such as in the case of chatbots or language translation.
Feature Engineering and Text Representation Techniques
Feature engineering involves selecting and transforming features (in this case, text data) to improve model performance. Text data often requires techniques like word embedding and TF-IDF (Term Frequency-Inverse Document Frequency) to convert text into numerical vectors that machine learning models can work with effectively.
Challenges and Solutions in Training ML Models with Text Data
Training machine learning models with text data comes with its unique set of challenges. These challenges include dealing with high-dimensional data, addressing class imbalances, and ensuring that models generalize well to unseen data. Solutions to these challenges often involve careful preprocessing, feature engineering, and selecting appropriate algorithms.
The Future of AI and Text Data
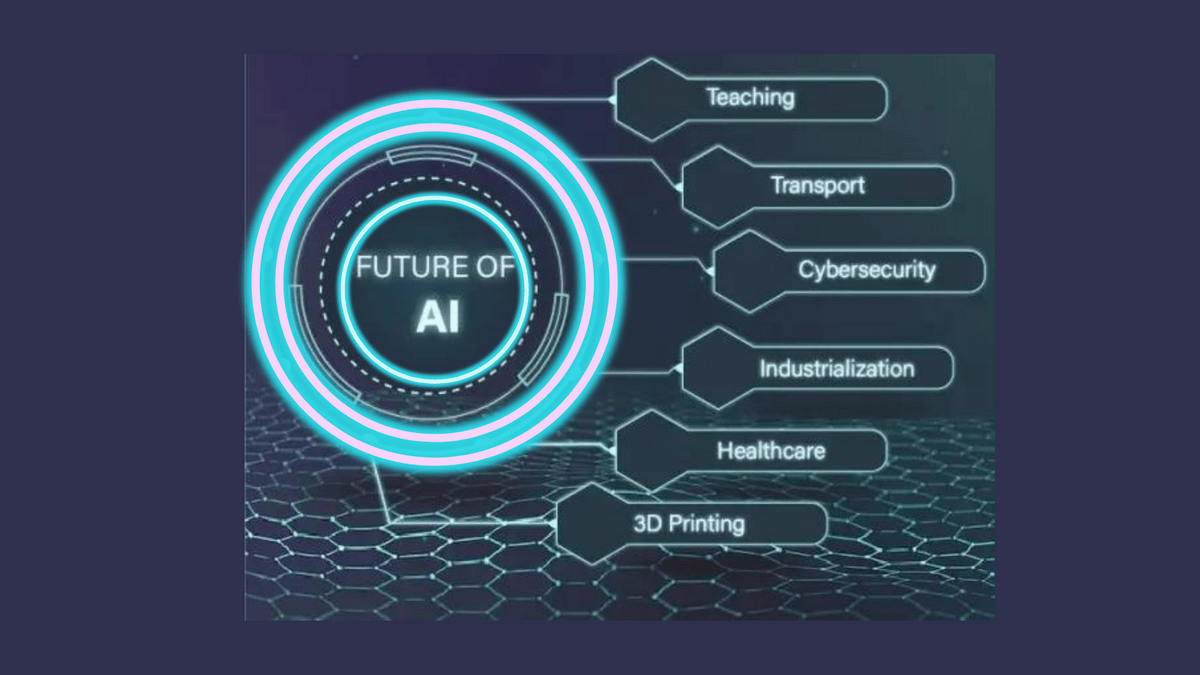
Our journey has taken us through the past and present of text data in AI. But what about the future?
Advancements in Text Data Collection and Analysis
The future holds the promise of more advanced text data collection techniques. Machine learning models will become more adept at understanding context and nuance, which will lead to even more accurate and personalized AI applications.
The Ethical Considerations of AI and Text Data
As AI and text data play an increasingly central role in our lives, ethical considerations become even more critical. Privacy, fairness, and accountability will remain key areas of concern. Safeguarding the responsible use of text data in AI will be an ongoing journey.
Conclusion:
As we conclude our journey into the world of AI-enhanced machine learning through text data, it becomes evident that text data is a dynamic and vital force in the evolution of AI applications. From sentiment analysis and chatbots to recommendation systems and language translation, text data's influence is pervasive and transformative.
Our journey continues, and the adventure is bound to be nothing short of remarkable.
Text Data Collection With GTS Experts
The journey towards AI success is paved with data, and in the domain of NLP, comprehensive text data is the cornerstone. Globose Technology Solutions Pvt Ltd (GTS) recognises the vital role of Text Data Collection in shaping the capabilities of AI models. As technology evolves and AI becomes more intertwined with daily life, the significance of language comprehension will only grow. GTS stands ready to drive this evolution by delivering comprehensive text datasets that empower AI to navigate the complexity of language with precision and insight.